Over the past few weeks we’ve been exploring aspects related to the new EU data protection law (GDPR) which will come into effect in 2018.
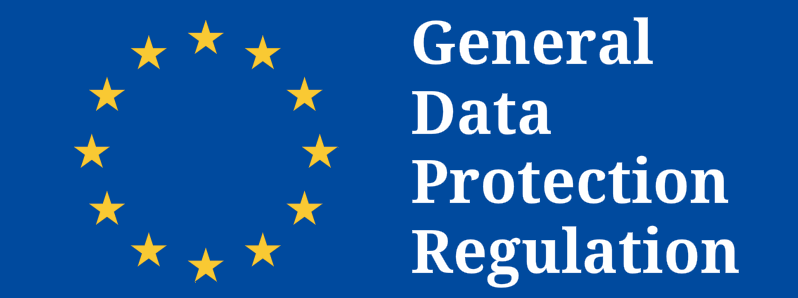
Over the past few weeks we’ve been exploring aspects related to the new EU data protection law (GDPR) which will come into effect in 2018.
The amount of data in the world is staggering. And as more and more organizations adopt digitally orientated business strategies the total keeps climbing. Modern organizations need to be equipped to manage Any2 – any data, anywhere.
Analysts predict that the total amount of data in the world will reach 44 zettabytes by 2020 – one zettabyte = 44 trillion gigabytes. That’s an incredible feat in and of itself. But considering the fact that the total had only reached 4.4 zettabytes in 2013, the rate at which data is collected and stored becomes even more astonishing.
However, it is equally incredible that less than 0.5% of that data is currently analyzed and/or utilized effectively by the business.
Perhaps the most obvious answer is opportunity. You likely wouldn’t be reading this blog if you weren’t at least passively aware of the potential insight that can be derived from a series of ones and zeros.
Start-ups such as Uber, Netflix and Airbnb are perhaps some of the best examples of data’s potential being realized. It’s even more apparent when you consider these three organizations refer to themselves as technology companies, as opposed to the fields their services fall under.
But with data’s potential, potentially open for any business to invest in, action, and benefit from, competition is more fierce than ever, which brings us to what else this new wave of data means for business. That being effective data management.
All of this new data is being created, or even stored, under one manageable umbrella. It’s disparate, it’s noisy, and in its raw form it’s often useless. So to uncover data’s aforementioned potential, businesses must take the necessary steps to “clean it up”.
That’s what the Any2 concept is all about. Allowing businesses to manage, govern and analyse any data, anywhere.
The first part of the Any2 equation, pertains to Any Data.
Managing data requires facing the challenges that come with the ‘three Vs of data’: volume, variety and velocity, with volume referring the amount of data, variety to its different sources, and velocity the speed in which it must be processed.
We can stretch these three Vs to five when we include veracity (confidence in the accuracy of the data), and value.
Generally, any data concerns the variety ‘V’, referring to the numbered and disparate potential sources data can be derived from. But as we need to be able to incorporate all of the varying forms of data to accurately analyze it, we can also say any data concerns the volume, and velocity too – especially where Big Data is considered.
Big Data initiatives increase the volume of data businesses have to manage exponentially, and to achieve desired time to market, it must be processed quickly (albeit thoroughly), too.
Additionally, data can be represented as either structured or unstructured.
Traditionally, most data fell under the structured label. Data including business data, relational data, and operational data, for example. And although the different types of data were still disparate, being inherently structured within their own vertical still made them far easier to manage, define, and analyze.
Unstructured data, however, is the polar opposite. It’s inherently messy and it’s hard to define, making both reporting and analysis potentially problematic. This is an issue many businesses face when transitioning to a more data-centric approach to operations.
Big data sources such as click stream data, IoT data, machine data and social media data all fall under this banner. All of these sources need to be rationalized and correlated so they can be analyzed more effectively, and in the same vain as the aforementioned structured data.
The anywhere half of the equation is arguably also predominantly focused on the variety ‘V’ – but from a different angle. Anywhere is more concerned with the differing and disparate ways and places in which data can be securely stored, rather than the variety in the data itself.
Although an understanding of where your data is has always been a necessity, it’s now become more relevant than ever. Prior to the adoption of cloud storage and services, data would have to have been managed locally, within the “firewall”.
Businesses would still have to know where the data was saved, and how it could be accessed.
However, the advantages of storing data outside of the business have become more apparent and more widely accepted. This has seen many businesses take the leap and invest in varying capacities, into-cloud based storage and software-as-a-service (SaaS).
Take SAP, for example. SAP provides one solution and one collated database, in favour of a business paying installation and upkeep fees for multiple softwares and databases.
And we still need to consider the uptick in the amount of businesses that buy customer data.
All of this data still has to be integrated, documented and understood in order for it to be useful, as poor management of data can lead to poor results – or, garbage in, garbage out for short.
Therefore, the key focus of the anywhere part of the equation is granting businesses the ability to manage external data at the same level as internal.
Effectively managing data anywhere, requires data modeling, business process and enterprise architecture.
Data modeling is needed to establish what you have whether internal or external, and to identify what that data is.
Business Processes is required to understand how the data should be used and how it best drives the business.
Enterprise Architecture is useful as it allows a business to determine how best to leverage the data to drive value. It’s also needed to ensure the business has a solid enough architecture to allow for this value to come to fruition, and in analyzing/predicting the impact of change, so that value isn’t adversely affected.
The best way to effectively manage Any Data, Anywhere, so that we can ensure investing in data management and analysis adds value, is to consider the ‘3Vs’ in relation to the data timeline. You should also consider the various initiatives (Data Modeling, Enterprise Architecture and Business Process) that can be actioned at each stage to ensure the data is properly processed and understood.
Any2 approach helps you:
For more Data Modeling, Enterprise Architecture, and Business Process advice follow us on Twitter and Linkedin to stay updated with the new posts!
By now many businesses would have already heard about the new General Data Protection Regulation legislation. But knowing is only half the battle. That’s why we’ve put together this GDPR guide.
Instead of utilizing built for purpose data management tools, businesses in the early stages of a data strategy often leverage pre existing, make-shift software.
However, the rate in which modern businesses create and store data, means these methods can be quickly outgrown.
In our last post, we looked at why any business with current, or future plans for a data-driven strategy need to ensure a strong data foundation is in place.
Without this, the insight provided by data can often be incomplete and misleading. This negates many of the benefits data strategies are typically implemented to find, and can cause problems down the line such as slowing down time to markets; increasing the potential for missteps and false starts; and above all else, adding to costs.
Leveraging a combination of data management tools, including data modeling, enterprise architecture and business processes can ensure the data foundations are strong, and analysis going forward is as accurate as possible.
For a breakdown of each discipline, how they fit together, and why they’re better together, read on below:
This post is part two of a two part series. For part 1, see here.
Data Modeling
Effective Data Modeling helps an organization catalogue, and standardize data, making the data more consistent and easier to digest and comprehend. It can provide direction for a systems strategy and aid in data analysis when developing new databases.
The value in the former is that it can indicate what kind of data should influence business processes, while the latter helps an organization find exactly what data they have available to them and categorize it.
In the modern world, data is a valuable resource, and so active data modeling in order to manage data, can reveal new threads of useful information. It gives businesses a way to query their databases for more refined and targeted analysis. Without an effective data model, insightful data can quite easily be overlooked.
Data modeling also helps organizations break down data silos. Typically, much of the data an organization possesses is kept on disparate systems and thus, making meaningful connections between them can be difficult. Data modeling serves to ease the integration of these systems, adding a new layer of depth to analysis.
Additionally, data modeling makes collaborating easier. As a rigorous and visual form of documentation, it can break down complexity and provide an organization with a defined framework, making communicating and sharing information about the business and its operations more straightforward.
Enterprise Architecture
Enterprise Architecture (EA) is a form of strategic planning used to map a businesses current capabilities, and determine the best course of action to achieve the ideal future state vision for the organization.
It typically straddles two key responsibilities. Those being ‘foundational’ enterprise architecture, and ‘vanguard’ enterprise architecture. Foundational EA tends to be more focused on the short term and is essentially implemented to govern ‘legacy IT’ tasks. The tasks we colloquially refer to as ‘keeping on the lights’.
It benefits a business by ensuring things like duplications in process, redundant processes, and unaccounted for systems and shelfware don’t cost the business time and money.
Vanguard enterprise architects tend to work with the long term vision in mind, and are expected to innovate to find the business new ways of reaching their future state objectives that could be more efficient than the current strategy.
It’s value to a business becomes more readily apparent when it enterprise architects operate in terms of business outcomes, and include better alignment of IT and the wider business; better strategic planning by adding transparency to the strategy, allowing the whole business to align behind, and work towards the future objective; and a healthier approach to risk, as the value (reward) in relation to the risk can be more accurately established.
Business Process
Business process solutions help leadership, operations and IT understand the complexities of their organizations in order to make better, more informed and intelligent opinions.
There are a number of factors that can influence an organization who had been making it by without a business process solution, to implement the initiative. Including strategic initiatives – like business transformation, mergers and acquisitions and business expansion; compliance & audits – such as new/changing industry regulations, government legislation and internal policies; and process improvement – enhancing financial performance, lowering operating costs and polishing the customer experience.
We can also look at the need for business process solutions from the perspective of challenges it can help overcome. Challenges including the complexities of a large organization and international workforces; confusion born of undefined and undocumented processes as well as outdated and redundant ones; competitor driven market disruption; and managing change.
Business process solutions aim to tackle these issues by allowing an organization to do the following:-
The Complete, Agile Foundation for the Data-Driven Enterprise.
As with data, these three examples of data management tools also benefit from a more fluent relationship, and for a long time, industry professionals have hoped for a more comprehensive approach. With DM, EA and BP tools that work in tandem with, and complement one another inherently.
It’s a request that makes sense too, as although all three data management tools are essential in their own right, they all influence one another.
We can look at acquiring, storing and analyzing data, then creating a strategy from that analysis’ as separate acts, or chapters. And when we bring the whole process together, under one suite, we effectively have the whole ‘Data Story’ available to us in a format we can analyze and inspect as a whole.
The countdown has begun to one of the biggest changes in data protection, but how much do you know about GDPR? In a series of articles throughout February we will explain the essential information you need to know and what you need to be doing now.
It stands for General Data Protection Regulation and it’s an EU legal framework which will apply to UK businesses from 25 May 2018. It’s a new set of legal requirements regarding data protection which adds new levels of accountability for companies, new requirements for documenting decisions and a new range of penalties if you don’t comply.
It’s designed to enable individuals to have better control of their own personal data.
While the law was ratified in 2016, countries have had a two-year implementation period which means businesses must be compliant by 2018.
The changes to data protection will be substantial as will be the penalties for failure to comply. It introduces concepts such as the right to be forgotten and formalises data breach notifications.
GDPR will ensure a regularity across all EU countries which means that individuals can expect to be treated the same in every country across Europe.
For processing personal data to be legal under GDPR businesses need to show that there is a legal basis as to why they require personal data and they need to document this reasoning.
GDPR states that personal data is any information that can be used to identify an individual. This means that, for the first time, it includes information such as genetic, mental, cultural, economic or social information.
To ensure valid consent is being given, businesses need to ensure simple language is used when asking for consent to collect personal data. Individuals must also have a clear understanding as to how the data will be used.
Furthermore, it is mandatory under the GDPR for businesses to employ a Data Protection Officer. This applies to public authorities and other companies where their core activities require “regular and systematic monitoring of data subjects on a large scale” or consist of “processing on a large scale of special categories of data”.
Data Protection Officers will also be required to complete Privacy Impact Assessment and give notification of a data breach within 72 hours.
At this stage it is unknown how the UK exiting the European Union will affect GDPR. However, with Article 50 yet to be triggered – the exit from the European Union is still over two years away and as such the UK will still be part of the EU in 2018. This means that businesses must comply with GDPR when it comes into force.
Penalties for failing to meet the requirements of GDPR could lead to fines of up to €20 million or 4% of the global annual turnover of the company for the previous year, whichever is higher. This high level of financial penalty could mean could have a serious impact on the future of a business.
Over the coming month, we will continue this series looking at how to get started preparing for GDPR now, why you need a Data Protection Officer and how GDPR will affect your international business.
Forget everything that you may have heard or read about enterprise architecture.
It does not have to take too long or cost too much. The problems are not with the concept of enterprise architecture, but with how it has been taught, applied and executed. All too often, enterprise architecture has been executed by IT groups for IT groups, and has involved the idea that everything in the current state has to be drawn and modeled before you can start to derive value. This approach has caused wasted effort, taken too long to show results, and provide insufficient added value to the organization.
In short, for many organizations, this has led to erosion in the perceived value of enterprise architecture. For others, it has led to the breakup of enterprise architecture groups, with separate management of the constituent parts– business architecture, information architecture, solutions architecture, technical architecture and in some cases, security architecture.
This has led to fragmentation of architecture, duplication, and potential sub-optimization of processes, systems and information. Taking a business outcome driven approach has led to renewed interest in the value enterprise architecture can bring.
But such interest will only remain if enterprise architecture groups remember that effective architecture is about enabling smarter decisions.
Enabling management to make those decisions more quickly, by having access to the right information, in the right format, at the right time.
Of course, focusing on future state first (desired business outcome), helps to reduce the scope of current state analysis and speed up the delivery of value. This increases perceived value, while reducing organizational resistance to architecture.
This blog post is an extract taken from Enterprise Architecture and Data Modeling – Practical steps to collect, connect and share your enterprise data for better business outcomes. Download the full ebook, for free, below.
Donna Burbank’s recent Enterprise Management 360 podcast was a hive of useful information. The Global Data Strategy CEO sat down with Data Modeling experts, and discussed the benefits of Data Modeling, and why practice is now more relevant than ever.
You can listen to the podcast on the Enterprise Management 360 website here – and below, you’ll find part 2 of 2. If you missed Part 1, find it here.
Guest Speakers:
Danny Sandwell, Product Manager at erwin (www.erwin.com)
Simon Carter, CEO at Sandhill Consultants (www.sandhill.co.uk)
Dr. Jean-Marie Fiechter, Business Owner Customer Reporting at Swisscom (www.swisscom.ch)
Hosted by: Donna Burbank, CEO at Global Data Strategy (www.globaldatastrategy.com)
Do you think that a Data Model is both a cost saver and revenue driver? Is data driving business profitability?
Simon Carter
Yes, I think it does. Data models obviously facilitate efficiency improvements, and they do that by identifying and eliminating duplication and promoting standardization. Efficiency improvements are going to bring you some cost reduction, and the reduction in operational risk through improved data quality can also deliver a competitive edge.
Opportunities for automation and rapid deployment of new technologies via a good understanding of your underlying data can make an organization agile, and reduce time-to-market for new products and services. So overall, absolutely, I can see it driving efficiency and reducing costs and delivering serious financial benefit.
Jean-Marie
For us, its mostly something that increases efficiency and in the end help us reduce costs, because it makes maintenance of the data warehouse much easier. If you have a proper data model you don’t have that chaos that happens if you just get data in and never model it correctly.
To start with its sometimes easier to just get data in and get the first reports out, but down the road when you try to maintain that chaos, it is much more costly. We like to do the thing right from the beginning; we model it, we integrate it, we avoid data redundancy that way, and it makes it much cheaper in the end.
You have a little bit longer at the beginning to do it properly, but in the long-run or medium-run you’re much more efficient and much faster. Because sometimes, you already have the data that is needed for a new report, and if you don’t have a data model, you don’t realize that you already have that data. You recreate a new interface and get the data a second, third, fourth or tenth time, and that takes a lot longer and is more costly.
So yes, it’s certainly more efficient, reduces costs, and because you have the data and can visualize what you already have, it certainly gives some more opportunity to get new business or new ideas, new analytics. That helps the business get ahead of the competition.
Danny Sandwell
What I’m seeing is a lot surveys of organizations, whether it’s the CIO, CEO or the CDO, and talking about their approach to data management and their approach to their business from a data-driven perspective. There’s an unbelievable correlation between people that take a business-focused approach to data management, so business alignment first, technology and infrastructure second, and the growth of those companies overall.
Businesses that are a little behind the curve in terms of that alignment tend to be lower-growth companies. And the way that people are looking at data – because they’re looking at it as a proper asset, they’re not just looking at return-on-investment, they’re looking at return-on-opportunity – increases significantly when you bring that data to the business and make it easy for them to access.
So, the data model is the place where business aligns with data, so to have a business-driven data strategy requires that process of modeling it properly. And then pushing that information out. One of the benefits of data modeling, it doesn’t just support the initiative today, if you do it right and set it up right, it supports the initiative today, tomorrow and the day after that, at a much lower cost every time you iterate against that data model to bring value to a specific initiative.
By opening it up and allowing people to understand the data model in their own time and in their own terms, it increases trust in data. And when you have trust in data, you have strategic data usage. And when you have strategic data usage, all the statistics are showing that it leads to not just efficiencies and lower costs, but to new opportunities and growth in businesses.
Who in an organization typically uses Data Models?
Jean-Marie
In our organization, the users are still a majority of technical users. The people that work either in Business Intelligence, building analytics, building reports, or building ETL jobs and stuff like that. But increasingly, we also have power users that are outside of Business Intelligence that are sufficiently technical enough that they can see the use of the model.
They use the model as documentation to see what kind of data they need for a report, cockpit, BI, and how to link that data together to get something that is efficient and meaningful. So, it’s still very technical at Swisscom but it’s getting a little bit broader.
Danny Sandwell
I think for a large segment of the business world, it is still the technical or IT person. The viewing and understanding and more collaboration is on the business-side. But I think there’s also a difference in terms of the maturity of the organization and the lifecycle of the organization.
Organizations that have a large legacy and have been transitioning from a brick-and-mortar traditional business to more of a digital business, they have some challenges with legacy infrastructure, and the legacy infrastructure requires IT being involved. A lot more hands on, because it’s just that big and complex and there are a lot constraints.
You have a lot of organizations that are starting up now that have no legacy to deal with and have access to the cloud and all these self-service, off-premise type capabilities, and their infrastructure is much newer. And what I’m seeing in organizations like that, is beyond just viewing data models, they’re actually starting to build the data models.
So, your starting to see power-users or analysts on the business-side, and folks like that who are building a conceptual data model and then using that model to start going to whatever IT service they have, whether in the cloud or on-premise, to show what their requirements are and have them have those things built underneath.
So, we’re still very much in flux in terms of where an organization is, what their history is and how fast they’ve transformed in terms becoming a digital business. But I’m seeing the trend where you have more and more business people involved in the actual building at the appropriate level, and then using that as the hand-off and contract between them and all the different service providers that they might be taking advantage of.
Whether its traditional and ETL type architectures, or whether its these new analytics use-cases supported by data virtualization. At the end of the day, the business person is able to articulate their requirements and needs, and then push that down, where it used to be more of a bottom up approach.
Simon Carter
I very much follow the line that Danny was taking there, which is that most of the doing is still done by the technical team, most of the building of the models is done by the technical team. While a lot of looking at models is done by the business users, they’re also verifying things and contributing significantly to the data model.
I’ll go back to my common taxonomy. You know, business models are being used by business analysts to validate data requirements with subject matter experts, and can be the basis of data glossaries used throughout an organization.
Application models are used by solution architects who are designing and validating solutions to store and retrieve the business data, and communicate that design to developers. Implementation models are used by database designers and administrators to create and maintain the structures needed to implement design.
Increasingly though, the business-level metadata is being used to enable those business users to drive down into the actual data, and verify its lineage and quality. And that’s due to the ability to map a business term right through the various models to the data it describes.
With a lot of data-driven transformation being focused around new technologies like Cloud, Big Data and Internet of Things, is Data Modeling still relevant?
Simon Carter
I think data modeling is still incredibly relevant in this age of data technologies. Big Data is referring to data storage and retrieval technology, so the Business and Application models are unaffected. All we need is for the Implementation models to be able to properly represent any new technology requirement.
Danny Sandwell
Generally, there’s new technology that comes out and everybody thinks they are the be-all-end-all to basically re-engineer the world, and leave everything else behind. But the reality is, you end up using Big Data for the appropriate applications that traditional data doesn’t handle, but you’re not going to rip-and-replace all the infrastructure that you have underneath supporting your traditional business data.
So, we end up with a hybrid data architecture. And with that hybrid data architecture, it becomes even more important to have a data model because there are some significant differences in terms of where data may physically sit in the organizations.
You know, people get this new technology and they think they don’t need any models and they know how to work with the technology. And that works when things are very encapsulated, when things are together and they’re not looking for integration. But the reality is, whatever is in Big Data probably needs to be integrated with the rest of the data.
So, what we’re seeing is at the outset, people are using the data model to document what is in those Big Data instances, because it’s a bit of a black box to the business, and the business is where the data drives value – so the business requires it. And as we see more of an impetus for proper data governance, both to manage the assets that are strategic in our organization, but also to respond to the legislative and regulatory compliance requirements that are now becoming a reality for most businesses, there is a need there.
First off, it’s a documentation tool so that people can see data no matter where it sits, in the same format, and relate to that data from a business perspective. Its building it into the architecture so you can see that its governed and managed with the same rigor that the rest of your data is, so you can establish trust in data across your organization.
Jean-Marie
I think what we see at our place, when I look at the Big Data cluster that we have, it’s a mix. If you have stuff that you do, a one-time shot at the data and then some analytics, then you’re probably using something like schema on read and you don’t really have a data model.
But as soon as you get a Big Data cluster to do analytics in a repetitive way, where you have the same questions popping up every day, every week or every month, then you certainly will have some part of your Big Data cluster that are schema on write, and then you have a data model. Because that’s your only way that you can ensure that your analytics, data mining and what not always encounter the same structure of the data.
You have some parts of the Big Data cluster that are not modeled because they are very transient. And you have some parts that are used as a source for your data warehouse or other analytics systems. Those are definitely modeled, otherwise you waste too much time every time something changes.
For more Data Modeling insight, follow us on Twitter here, and join our erwin Group on LinkedIn.
Company mergers, even once approved, can often be daunting affairs. Depending on the size of the business, there can be hundreds of systems and processes that need to be accounted for, which can be difficult, and even impossible to do in advance.
Therefore, following a merger, businesses typically find themselves with a plethora of duplicate applications and business capabilities that eat into overheads, and make inter-departmental alignment difficult.
These drawbacks mean businesses have to ensure their systems are fully documented and rationalized. This way, the organization can comb through their inventory and make more informed decisions on which systems can and should be cut or phased out, in order for the business to operate closer to peak efficiency and deliver the roadmap to enable that change.
This is why enterprise architecture (EA) is essential in facilitating company mergers.
EA helps a business’ alignment throughout the organization, providing a business outcome perspective for IT, and guiding transformation. It also helps a business define strategy and models, improving interdepartmental cohesion and communication. Roadmaps can be leveraged in order to provide a common focus throughout the company, and if existing roadmaps are in place, they can be modified in order to fit the new landscape.
Finally, as alluded to above, EA will aid in rooting out duplications in process and operations, making the business more cost efficient on the whole.
The makeshift approach:
The first approach is more common in businesses with either no, or a low maturity enterprise architecture initiative. Smaller businesses often start out with this approach, as their limited operations and systems aren’t enough to justify real EA investment. Instead, businesses opt to repurpose tools they already have, such as the Office Suite.
This comes with it’s advantages that mainly play out on a short term basis, with the disadvantages only becoming apparent as the EA develops. For a start, the learning curve is typically smaller, as many people are already familiar with software, and the cost per license is relatively low when compared with built-for-purpose EA tools.
But as alluded to earlier, these short term advantages will be eclipsed overtime as the organizations EA grows. The adhoc, Office Tools approach to EA requires juggling a number of applications and formats, that can stifle its effectiveness. Not only do the operations and systems become too numbered to manage this way, the disparity between formats stops a business from performing any deep analysis. It also creates more work for the Enterprise Architect, as the disparate parts of the Office Tools must be maintained separately when changes are made, in order to make sure everything is up to date.
This method also increases the likelihood that data is overlooked as key information is siloed, and it isn’t always clear which data set is behind any given door, disrupting efficiency and time to market. It isn’t just data that siloed, though. The Office Tools approach can isolate the EA department itself, from the wider business. The aforementioned disparities aided to the mis-matching formats can make collaborating with the wider business more difficult.
The EA tool approach:
In essence, the EA tool approach is the polar opposite to Office Tools based EA. The disadvantages of implementing a dedicated EA tool tend to be uncovered in the short term. Such disadvantages include the cost and ease (or lack thereof) of installation.
But as an organization’s Enterprise Architecture grows, investing in dedicated EA tools becomes a necessity, making the transition just a matter of timing.
When implemented though, management of an organization’s EA becomes much easier. The data is all stored in one place, allowing for faster, deeper, and more comprehensive analysis and comparison. Collaboration also benefits from this approach, as having everything housed under one roof makes it far easier to share with stakeholders, decision makers, C-Level executives and other relevant parties.
Considering all of this, the up side to investing in dedicated EA tools become more apparent. A dedicated EA tool will help an organization achieve the benefits of enterprise architecture to their full extent. Some organizations may still have reservations about cost, but thanks to SaaS-based EA offerings, the financial and time costs of implementing a new EA tool are minimized too.
The SaaS approach eliminates initial installation costs in favor of a more affordable, less binding, agility enabling pricing plan. This decreases the likelihood that the investment will become another piece of expensive shelfware. There are other benefits to the SaaS model too, including more frequent and less intrusive updates, and a global EA that’s updated for everybody in real time, and is accessible to all approved parties from anywhere in the world – as long as there’s an internet connection.
If Gartner’s word is anything to go by, Big Data adoption is seeing an uptick. The analyst cites “increasing inquiries” into Big Data analytics tools, as more businesses look for new opportunities in capturing increasing amounts, or eek more value out of the large amounts of data they already own.
Supporting this, a US-based study into budgetary plans, indicated that 60% of CIOs believe Big Data will be a ‘top driver’ of IT spending.
Generally speaking, a collective shift in the industry is rarely a coincidence. Trends are usually propped up by a series of concrete benefits, and in the case of Big Data, this is no different.
Companies with a well actioned Big Data strategy can make more well-rounded and informed decisions. One of the key uses of Big Data is to get a better understanding of the market, prospects and customers.
Data is sometimes referred to as the “oil of the 21st century”, and customer data specifically, is arguably the key factor in that. Online and digital business models, and notably Social Media, has opened up a two way dialogue between people and the rest of the world, and provided businesses with an unprecedented level of meaningful data insight.
As a result, businesses now know more about their customers than ever, and this information can be used to earn new ones.
In gaining a better understanding of the market, Big Data can be used to gauge potential market interest. As well as indicating whether a new service or product is worth providing, this information can also help businesses forecast supply with greater accuracy, in relation to demand.
As well as understanding external factors, Big Data can also provide new insights to understand internal operations and process efficiencies. The data can can highlight capabilities and processes that are ripe for improvement, and be used to guide the best course of action to optimization.
When businesses get it right, Big Data can open a lot of new doors, and allow a business to reach new heights. But simply collecting the data isn’t enough. To return to an aforementioned analogy, much like oil, Big Data isn’t of much use in its raw form. It needs to be refined, and concentrated into something decipherable, and greater than the sum of its parts.
Both data modeling (DM) and enterprise architecture (EA) are essential in making the most out of this refinement process. Data Modelling helps you to analyze the data by providing a contextualized perspective of the information across various platforms. Enterprise Architecture helps you translate and apply data to strategic business and IT objectives. It also aids in indicating which data insights are a priority within your current-state organization and which data will be critical to support your future-state.
This is great news for businesses who already have established a functioning EA and/or DM initiative, but those behind in terms of architecture and modeling will have to find room in the budget for new tools.
In the past, this would have always been a daunting exercise. Encouraging stakeholder investment into EA especially has been notoriously difficult. High, local installation costs and long term contractual commitments are enough to make any business think twice, especially when the business is trying to stay agile. – and this goes doubly for a specialist profession such as EA, where business leaders and stakeholders might not be fully aware of the potential gains.
However, the introduction of Software as a Service based tools has provided the aforementioned apprehensive businesses a new life line. Local installation costs and long term commitments are avoided, in favor of flexibility.
What’s more, integrating enterprise architecture tools with data modeling tools brings significant benefits in alignment of processes and systems.
Businesses appear to have consensually agreed upon the value of a data-driven organization. Yet, studies have shown that very few businesses are maximizing the data opportunity. Senior Executives surveyed during an Economist Intelligence Unit (EIU) study, overwhelmingly missed out on “broad positive results”, with only 2% reaching this desired outcome.To put this into perspective, 70% of those surveyed indicated that data and analysis were high up on the organization’s agenda. So what’s going wrong?
There are multiple factors in play and the areas in which businesses need to improve are rarely black and white. However, one stand out approach to help make the most out of data, is a capable and efficient Enterprise Architecture initiative. Coupled with a robust Data Modelling strategy, data driven businesses should begin to thrive under the deeper insights offered by the approach.
Effective data management begins with capturing the structure and related metadata associated with your enterprise data assets. However, the native metadata available from most datasources is often cryptic. It’s also limited to a technical viewpoint, and very brittle in terms of providing a usable foundation for visibility, comprehension and analysis across the various stakeholders it concerns. These technical descriptors need to be augmented and integrated with additional viewpoints that describe the business purpose and usages of the data.
Historically, data modeling has been perceived as a design and deployment tool for creating databases. However, data models can deliver as much or more value and utility as a vehicle to discover, document, expose, analyze and govern data sources for the wide range of stakeholders involved in collaborative data management.
Data models are visual in nature, encapsulate multiple perspectives (conceptual, logical, physical) and enable the standardization of metadata to provide a consistent, contextual view of data across disparate platforms.
Additionally, data models allow organizations to de-couple from the production environment, enable scenario analysis and planning without risk to ongoing operations, and iteratively deploy “to-be” designs in an efficient and effective manner.
Being data-driven isn’t just about recording and examining as much data as possible. Businesses need to be active in how they leverage data, in order to provide insights into possible strategies going forward. But to be able to effectively leverage such data, businesses need to be smart about how data is stored, segmented and applied to decision-making.
The sheer volume of data, typically kept by data driven organizations can make it difficult to cipher through to what is relevant. This can give a false impression that the data being stored isn’t useful.
To streamline the data, making it more manageable and more insightful, businesses need to focus exclusively, on the data that’s relevant to the organization’s current aims, goals and objectives.
A well actioned Enterprise Architecture practice can achieve this, as a business’ current capabilities as well as its desired future state can be highlighted. From here, a business can work out what steps it can take, and which of those it will need to take in order to reach the desired future state. A good understanding here, should indicate which areas of data to drill down on.
Additionally, Enterprise Architecture tools supporting a View Manager can help further, still. Views are essentially a real-time snapshot of information, based on parameters decided by the user. Manipulating data into different views by changing the parameters can give an organization a better idea of what to prioritize (through sorting), but also give the organization a generally more manageable representation of data by removing everything that isn’t relevant.
In the section above, we talked about the merits of sifting through data, to de-clutter and help the information be deciphered more readily. But although data is far easier to understand in smaller, specific chunks, it needs to be whole in the first place to be truly accurate.
Many businesses cite wanting to improve communication across the organization – especially communication that happens between departments. Often, they want to improve communication in the literal sense – sharing ideas, updates and goals through some form of conversation. However, what some organizations are missing, is that these issues in communication are often rooted in misalignment of departments.
Businesses are forced into giving updates – verbal, written or otherwise – because the departments aren’t transparent enough for employees and other departments to see for themselves. Departments operate as siloed, independent areas of the business, instead of as smaller parts of one larger cohesive unit.
Data suffers because of this too. If data is fragmented across different departmental silos that aren’t implicitly open to one another, the data will never show the full picture.
Enterprise Architecture works to align these departments by instilling a common perspective of the current state of the architecture and wider business, as mentioned above. But it also helps align the business in its processes and systems, by highlighting areas of duplication (where two systems/processes are used in place of one), enabling the organization to move to one system, and unify the back end data contained in both.